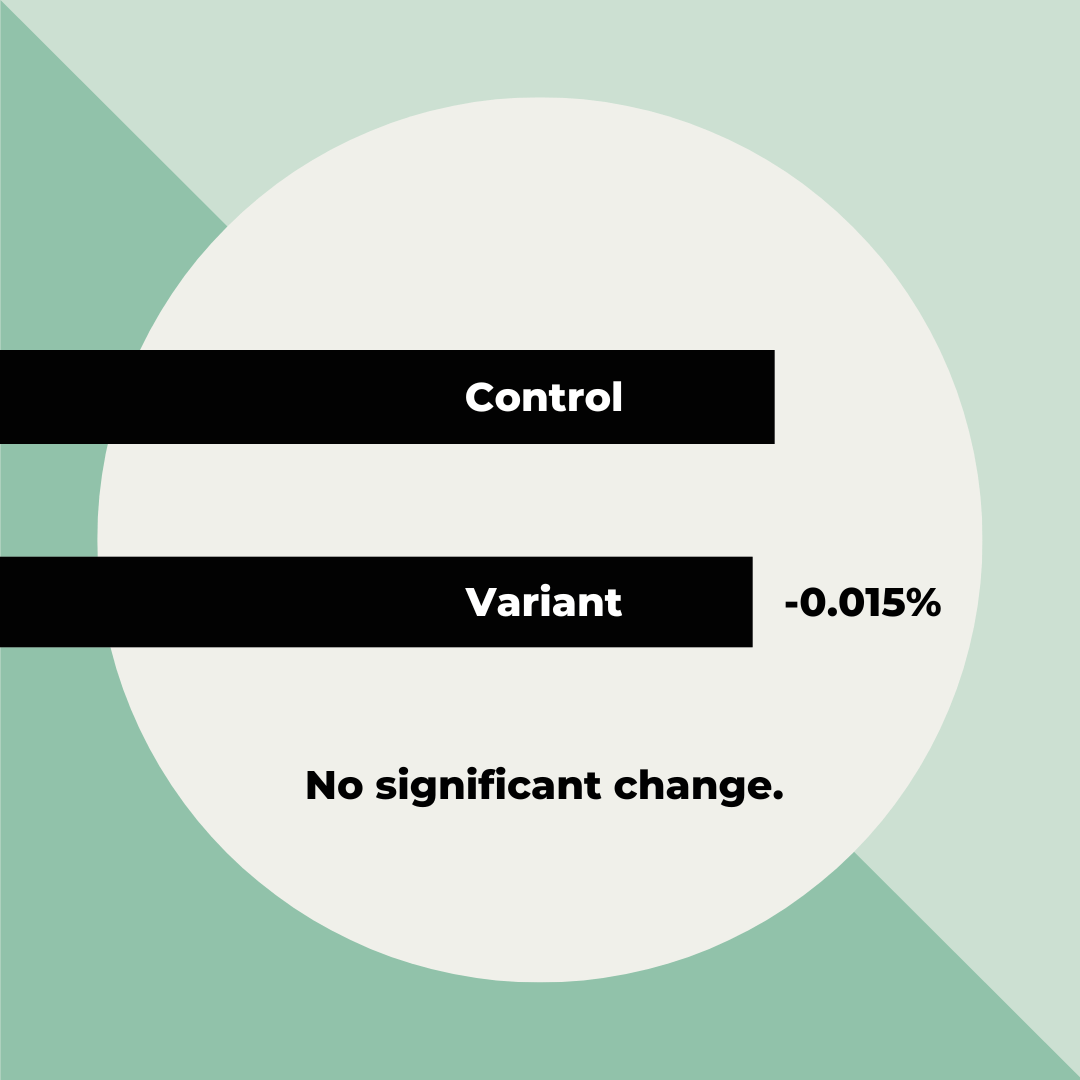
Publication: Nov 16, 2022
What if your A/B test results are not significant?
To get straight to the point: most experiments will have non-significant results. Even if you have looked closely at your MDE when designing an experiment and have a representative data set.
According to Experiment Engine data, 50% to 80% of test results are insignificant. VWO and Convert.com have made estimates showing that only about 1 in 7 A/B tests is a winning test.
What you can do if you have a non-significant test result:
First of all, you may be testing for non-inferiority: you hope that your change will have no impact, then of course you pass the test.
In addition to calculating your test by your main KPI, you may also have some supported metrics. Do they tell you something? Ideally, you have set these supporting goals before. In the case of increasing conversion for eCommerce, your main goal is often transactions or sales and a supporting goal is add to carts or CTR to your checkout.
If your test has run on both mobile and desktop, it is always good to see if there are major differences between these devices. Beware of data torture. Sometimes conversion specialists or data analysts are pushed towards data torture by managers or stakeholders. Questions can be asked such as: 'And have you measured this? And this? And this? There has to be a winner somewhere, right?” And yes: if you break your data into categories often enough or dig deep enough, there is always a winner to be found. But: that result is probably nowhere near your original metrics and what you wanted to measure. Often it is a distraction, to reassure ourselves that we have found 'something'.
Are you afraid that another test has influenced your A/B test? Segment this data from your test. Sometimes you can collect qualitative data (such as a hotjar/usabilla poll) in the variant of your test, or you can look at user recordings or heatmaps, do you learn anything from that?
Too many non-significant outcomes, what can you do?
If you doubt the number of experiments that have a non-significant outcome, improve your process:
Do you collect enough data and do you have a representative data sample? You can check this with the Speero Calculator. Look especially at the Minimal Detectable Effect. That is the effect you can measure with your data set. A 'healthy' MDE is < 15%. And as a guideline you can think of small copy tweaks, for that you need a lot of traffic and about 1 - 3% MDE, larger changes that can have a lot of impact can be estimated at an MDE of 1 - 10%. And innovation, disruptive ideas may have a greater impact.
Was your hypothesis well put together? Check out this Hypothesis Kit.
What data point did you use to support your hypothesis, or did the idea for the test come as someone's opinion or assumption? Check whether your research and the data from which your A/B test originated have not been misinterpreted, or whether the situation has changed in the meantime (if you have only collected data during Black Friday, then this data is of course very different from regular data). Or maybe you misjudged the input?
Can it be validated in another way? Think of User Testing, Copy Testing, 5 Second Testing.
Wasn't the change you made too small for the MDE (Minimum Detectable Effect)? Maybe you need to execute your idea or hypothesis in multiple places on the page or make your idea bigger?
Was the test fired at the right visitors? If your change was at the bottom of the page, make sure that the test is only fired at visitors who come to your website at that point (you can do this, for example, based on scroll percentage)
When do you improve and repeat your test?
Sometimes a change is not big or bold enough (for the dataset – MDE). You may want to make your change bigger or try the test in a different direction. Ultimately, prioritization helps with this. If you have a new idea after a non-significant test, a prioritization model such as PXL helps you determine whether you should get started right away, or whether other ideas have more potential.
The philosophy of accepting insignificant results
Let me tell you something personal: I thought many of my ideas, adjustments or tests would have a significant impact. But now that I've done 600+ experiments, I know better. Most implementations of ideas have only a small effect on visitors, an effect that often cannot be measured.
This taught me something about how, as humans, we overestimate the effect of our ideas, opinions, and adjustments. Most changes don't matter much at all. It reminded me of Illusory Superiority (a person overestimates his or her own qualities), but also the IKEA effect (the more time you spend setting something up, or coming up with an idea, the more you like it).
Many decisions I concluded, for A/B testing, in business or in your personal life don't have such a big effect at all. It might be 1 in 7 ideas that really blow your mind. And thanks to A/B testing: you can find it 1 in 7.
This blog was also published on Speero.com